Service-Meister - How grandcentrix brings Data Science, IoT and the handicraft together
The project is funded by the German Federal Ministry of Economics (BMWi) and executed by a collaboration of industry, research institutions and implementation partners, such as grandcentrix. The goal of Service-Meister is to bring artificial intelligence or, more generally speaking, data-driven decision-making processes to German SMEs to make them capable of competing in the future. The six speedboats, each consisting of an implementation partner and an industry partner, are currently developing data-based solution approaches for typical challenges faced by SMEs. These solutions will subsequently be specified into generic services and made available to German SMEs starting in 2022 as part of a service ecosystem for AI services.
As a provider of IoT solutions for intelligent products with its own Competence Center for Data Science, grandcentrix qualified as one of only three implementation partners in the project. The data science team, founded three years ago and consisting of five data scientists and engineers, convinced not only with a broad range of services, from statistical analysis and rapid prototyping to design, application and continuous training of machine learning models, but also with the pragmatic approach of using data as early as possible and as meaningfully as possible.
The speedboat by Würth and grandcentrix
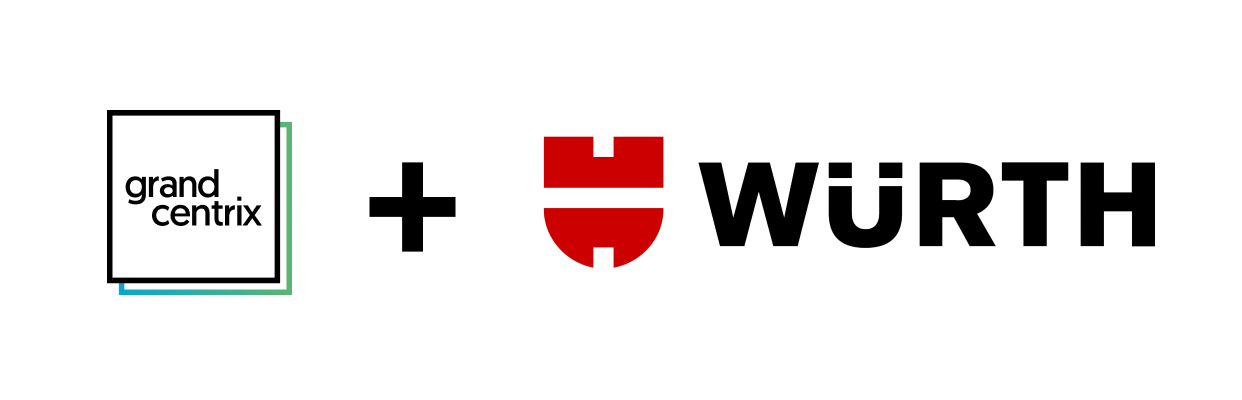
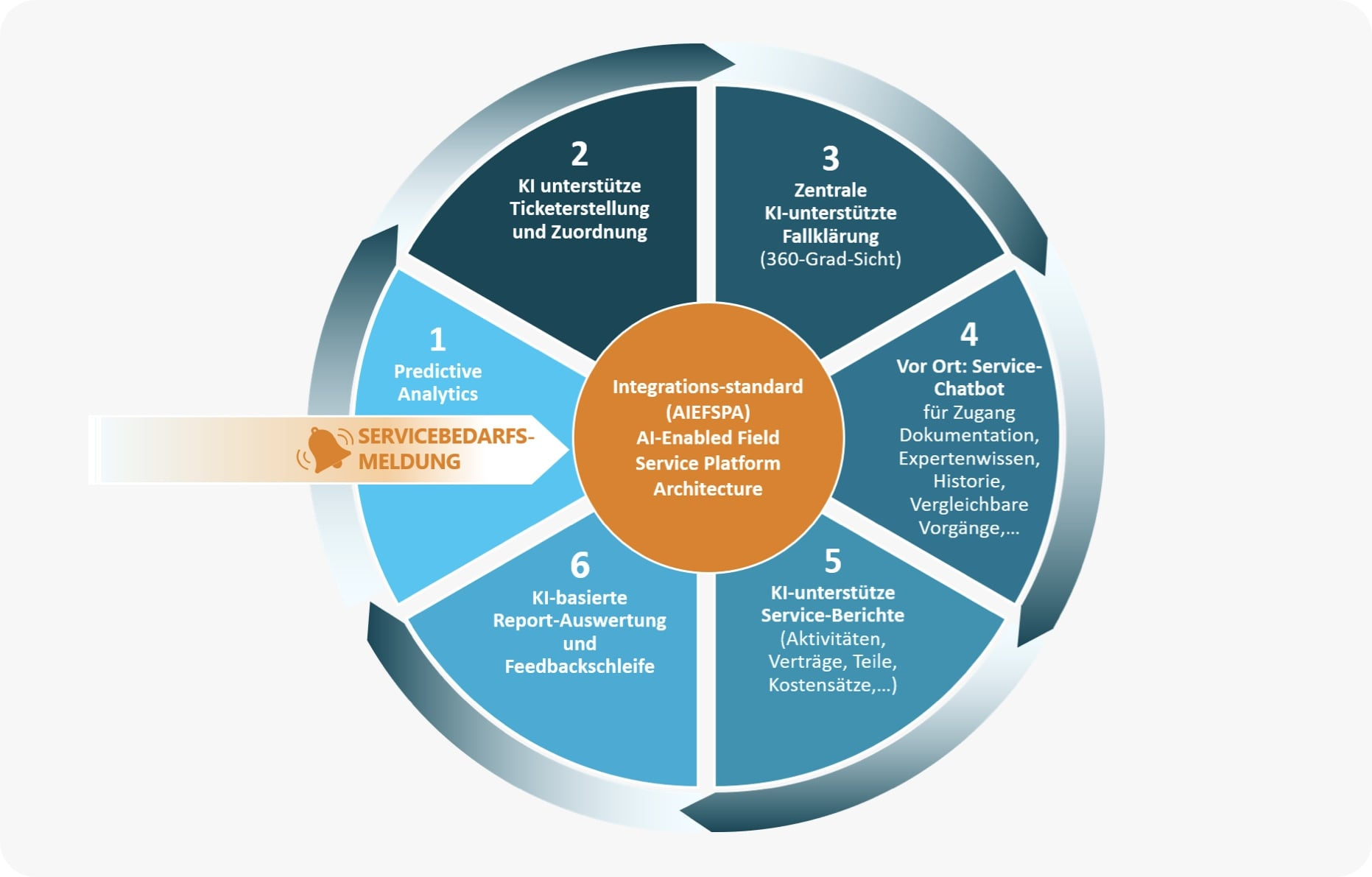
Initial findings and forecast
Besides a descriptive data analysis of the service cases, the focus last year was on the classification of service tickets. For this, different approaches, partly based on Natural Language Processing (NLP), were used. In addition, an Azure pipeline was built to identify significant anomalies in ratio metrics.
For the prediction of fault classes to fault descriptions of customers and based on evaluations of completed service tickets via NLP, Laura Ohrndorf successfully pursues the goal to accelerate processing and troubleshooting and to enable the planning of resources including possible pre-ordering of required spare parts. Here, the data is prepared with spaCy to extract the error descriptions from the tickets. A generalized linear model (GLM) is then trained based on this data to predict the defect classes.Thanks to Sean Mirchi’s Ratio Comparison Pipeline implemented using Azure ML Services and set up in the Azure Data Factory, questions such as whether the error rate differs significantly between tools can be answered. In addition, ratio metrics can be constantly monitored, allowing changes to be identified at an early stage. Since anomaly detection is currently still being trained with simulated data, it can be applied in a wide variety of areas in the future.
With collaboration across the Service-Meister consortium, the specification of generic services will begin this year, to be published with open-source licence to be freely available to SMEs (e.g. via GitHub). The platform is intended to support understanding without technical knowledge how and which challenges can be solved using data-based algorithms. In the process, generic end-to-end implementations are to be made available using concrete examples as a “proof of concept” and the great potential is to be demonstrated through visualizations and key figures.